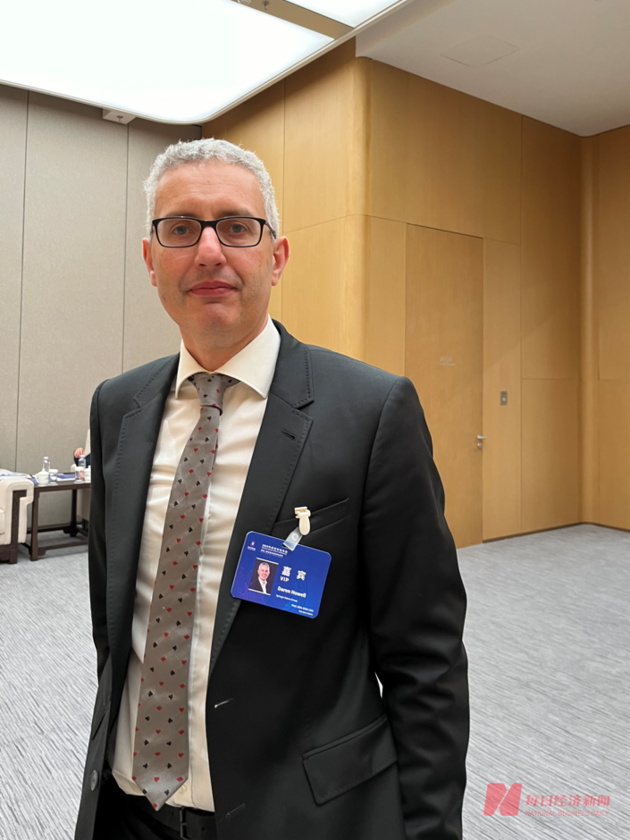
Photo/Shi Yuxi (NBD)
On April 28, 2024, the 2024 Zhongguancun Forum Annual Meeting held a parallel forum titled “Digitization Promoting Innovation in Large Enterprises: AI Empowering Industry Development” in Beijing.
During the forum, Daren Howell,Vice President of Research Intelligence at Springer Nature, delivered a speech analyzing how artificial intelligence research can be translated into industry impact. He emphasized that AI, as an innovation catalyst, continues to evolve. Starting with the analysis and structuring of vast knowledge, information, and data, AI reshapes information flow, enhances efficiency, drives innovation, integrates into real-world scenarios, and promotes industrial transformation.
The forum also featured an interview with Howell, focusing on the impact of AI on scientific research.
AI Hallucinations Can Be Controlled
NBD: Due to technical limitations, large language models can sometimes generate incorrect or misleading information, a phenomenon known as AI hallucinations. This can be particularly problematic in scientific research, where accuracy is paramount. Do you believe AI hallucinations can be resolved?
Howell: We have found that AI hallucinations can be controlled by providing large language models with accurate information and instructing them to base their responses on that information.
For example, if I read a book last year and you ask me about it today, I might get some details wrong. But if I have the book in front of me, I can refer to the pages and provide a detailed account. Similarly, by treating large language models in the same way, AI hallucinations can be largely controlled. However, a human should always review and take responsibility for the output, as this involves quality control and ownership issues.
NBD: Do you think this issue can be completely solved? Can we 100% trust the text generated by large models?
Howell: If you combine large language models with facts and ask them to retrieve those facts, then I think there is a solution. Large models will be as good as many people at remembering facts, but humans themselves cannot always remember everything accurately, so I think 100% trust is a high standard for large models.
NBD: In recent years, with the development of artificial intelligence, there has been an increase in academic misconduct such as using AI to write papers and falsify data. What is your view on this issue?
Howell: Plagiarism and other forms of academic misconduct have always existed, but the rise of large language models has made them more prevalent and easier to do. As a world-renowned publishing institution, we have always taken academic misconduct very seriously.
On the one hand, we have been using AI technology for text similarity checking and plagiarism detection for a long time, which has effectively curbed academic misconduct. On the other hand, when people publish articles, there is only one author, and the author has a reputation, which they also need to consider protecting.
Therefore, I would like to say to the authors, if you use AI in your articles, please make sure you are aware of it. Plagiarism, data falsification and other academic misconduct can be detected by our technology.
In fact, we have been using AI to detect plagiarism, academic misconduct, and falsification in papers for a long time. With the advancement of technology, we have recently made new breakthroughs in detecting meaningless or fake papers generated by AI.
For example, AI can be used to detect papers that are "nonsense" generated by large models. As mentioned above, when AI is used to generate text, it may generate meaningless text, and our methods can identify fake content generated by large models. In practice, we will also use manual review, but it is undeniable that AI can improve work efficiency and help us optimize solutions.
Key Issues in Developing Large Models of Scientific and Technological Literature
NBD: What are the key issues in developing large models of scientific and technological literature?
Howell: Data and computation are two critical aspects.
In terms of innovation, some fields have high-quality data suitable for AI. For example, we can use language models to learn the functional characteristics of proteins, but we haven't seen such significant progress in materials because the chemical structure of materials is not suitable for large language models.
I think this may be solved in the future because once you have a lot of training, you have data that you can predict. Through a lot of computation, algorithms can make real progress, and new algorithms will emerge.
NBD: How is AI changing the field of scientific research?
Howell: Many people spend less time writing, or they can write in different languages, not just their first language, or even code, such as computer code, which makes them more efficient in their workflows.
Industry leaders have a lot of data and powerful computing power, and they can combine these two things to create many innovations that were difficult to achieve in the past. In the past, there was too much data for one person to understand, but large language models and other types of AI can use all of this information to innovate.
NBD: The challenge we face is not only understanding innovation but also accurately measuring it. How do you think innovation should be measured?
Howell: I think the standard for measuring innovation is impact. Innovation is about how we can have a better environment, how we can cure different diseases, how we can improve transportation, how we can reduce energy use or create energy.
For our publishing institution, the standard for measuring impact is how scientific innovation benefits society and humanity. For example, you can see that the treatment of certain diseases has improved, and through research and innovation, the success rate of cancer treatment has improved. This can be used to measure the impact of scientific innovation.