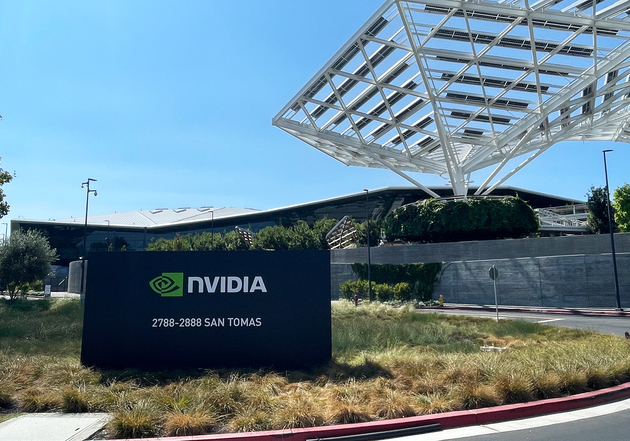
Photo/Zheng Yuhang (NBD)
In this era driven by data and computing power, NVIDIA has nearly monopolized the AI chip market with its high-performance GPU chips. However, as AI competition intensifies and chip supply becomes scarce, tech giants including Meta, Google, and Amazon have begun to explore self-developing chips.
On April 10th, local time, Meta announced the latest version of its self-developed chip, MTIA. The MTIA is a custom chip series designed by Meta specifically for AI training and inference tasks. The day before, Google also announced the launch of Axion, a data center chip based on Arm architecture.
The tech giants’ foray into chip development is driven not only by the desire to reduce supply dependency but also by cost pressures. Taking NVIDIA’s star chip product H100 as an example, its current price has soared to $25,000 to $30,000. According to Meta’s plan to acquire 350,000 H100s by the end of the year, the cost of these chips would be at least $8.75 billion.
Will their entry shake NVIDIA’s dominant position in the chip market?
Tech companies are starting to reduce reliance on NVIDIA
On April 10th, local time, Meta announced the launch of the latest version of its self-developed chip, MTIA v2, designed specifically for the ranking and recommendation systems of Meta’s social software. Early test results show that the performance of the latest version has significantly improved, being three times that of the first-generation AI inference accelerator MTIA v1 announced in May last year.
The day before, Google also announced the launch of Axion, a data center chip based on Arm architecture. According to Google, the performance of the Axion chip is 30% higher than that of general ARM chips, 50% higher than the current generation of x86 chips produced by Intel, and 60% more energy-efficient. Google plans to use Axion for a variety of services under Google, such as YouTube ad placement, big data analysis, etc.
In fact, in addition to Meta and Google, Microsoft, Tesla, and Amazon have also successively released custom chips capable of handling AI tasks.
There has also been much speculation about OpenAI’s self-developed AI chips. In January this year, Bloomberg cited insiders saying that OpenAI CEO Sam Altman is planning to use billions of dollars in funding to establish a semiconductor foundry of a certain scale.
According to analysts from market research firm CFRA, large tech companies are facing pressure on chip costs and need to alleviate it by self-developing chips. According to Fortune magazine, for large tech companies with funds, self-developed chips also help reduce dependence on external chip manufacturers such as NVIDIA and Intel, while also allowing companies to customize hardware based on their own AI models.
With the release of the top AI tool ChatGPT, the generative AI market has also triggered a race among major tech companies, with computing power being the core engine behind the booming development of this field.
Against this backdrop, high-performance GPU chips such as NVIDIA’s A100, H100, A800, and H800 have become the objects of competition among major AI companies, especially the H100. Last July, foreign media reported that NVIDIA’s H100 GPU for AI computing was in short supply. At that time, the delivery time for NVIDIA GPUs was as long as 11 months, and most NVIDIA customers had to wait nearly a year to receive their ordered GPUs. However, the supply bottleneck has now eased.
And naturally, such “hot cakes” come at a high price. It is reported that the price of the H100 has now soared to $25,000 to $30,000.
It is said that to build a supercomputer supporting OpenAI projects, Microsoft has invested hundreds of millions of dollars to connect tens of thousands of Nvidia A100 chips on the Azure cloud computing platform. It is estimated that even just to maintain the basic operation of ChatGPT, it would cost about $16 billion per year.
In March this year, Meta also announced the details and roadmap of its AI infrastructure layout, stating that it plans to acquire about 350,000 H100 GPUs from NVIDIA by the end of this year. By then, the company’s total computing power will be close to that provided by 600,000 H100s. Even at the lowest selling price of $25,000, the cost of these 350,000 H100s would be about $8.75 billion.
Is NVIDIA’s dominant position no longer existent?
According to The New York Times, NVIDIA sold 2.5 million chips last year, nearly monopolizing the market. According to data from research company Omdia, NVIDIA’s sales accounted for more than 70% of the entire market. Driven by strong chip demand, NVIDIA’s stock price rose by about 240% last year, and since the beginning of 2024, its stock price has risen by more than 83%, closing at $881.86 per share on Friday.
Some analysts believe that NVIDIA’s leading position may be threatened as tech giants enter the chip field. “Wood Sister” Cathie Wood pointed out in a recent interview with Barron’s Weekly that competitors such as Google, Amazon, Microsoft, and Tesla are all personally developing chips, which will drag down NVIDIA’s future revenue growth.
Alvin Nguyen, a senior analyst at consulting firm Forrester, believes that “although the chips designed by companies such as Google, Meta, and Amazon may not be as powerful as NVIDIA’s top products, this may benefit these companies. Especially, the waiting time for shipments will be shorter.”
“From Meta’s perspective, this provides them with a bargaining chip to negotiate with NVIDIA,” said Edward Wilford, an analyst at tech consulting firm Omdia. “They have other options besides NVIDIA.”
In addition, Nguyen also believes that self-developed chips can better adapt to the specific AI platforms of tech companies, thereby improving efficiency and saving costs by eliminating unnecessary functions. This means that although NVIDIA GPUs perform well in AI data centers, as a general-purpose hardware, they may not be as effective as custom chips in certain workloads and models.
However, the development of AI chips by tech companies is a long-term process. Nguyen expects that the development of these chips will take about a year and a half, and it may take several months before they can be implemented on a large scale. In the foreseeable future, the entire AI field will continue to rely heavily on NVIDIA to meet its computing hardware needs.
Moreover, self-developing chips does not mean that tech giants can completely get rid of their dependence on NVIDIA.
In fact, tech giants making chips is not a new thing. Google has been rolling out its self-developed AI tensor processing units since 2016, and Amazon Web Services announced the launch of its self-developed chip Trainium for training AI models in 2020. However, until the explosion of generative AI, only NVIDIA has been the leader in AI chips, becoming the biggest “shovel seller.”
Fortune reports that this is partly due to the manufacturing limitations of tech giants; on the other hand, NVIDIA has built the largest chip system, offering higher performance and compatibility with a wider range of software. The head of Amazon’s chip business once said in an interview with foreign media, “NVIDIA has excellent chips, but more importantly, they have an incredible ecosystem. Based on this, it is very challenging to get the market to accept a new chip.”
Data from research company Gartner shows that by 2027, the chip market is expected to more than double, reaching about $140 billion. Not only the providers of AI models are eyeing this cake, but well-known chip manufacturers such as AMD and Intel are also accelerating the launch of better-performing AI chips to challenge NVIDIA’s dominant position.
NVIDIA will naturally not sit idly by. In 2023, NVIDIA launched its own cloud service, where enterprises can use its chips. In addition, the company is cooperating with cloud providers such as CoreWeave based on its chip business, in order to compete with Amazon, Google, and Microsoft.
Disclaimer: The content and data of this article are for reference only and do not constitute investment advice. Verify before use.