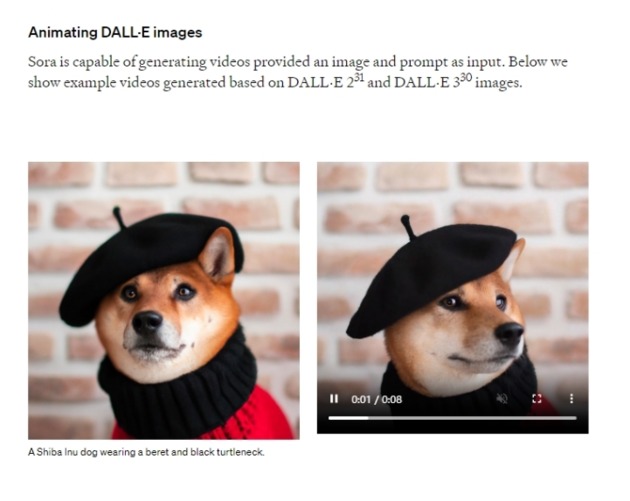
Photo/ Sora technical report
On February 16, OpenAI’s AI video model Sora made a stunning debut, generating videos that amazed people with their clarity, coherence, and timeliness. For a while, comments like “Reality doesn’t exist anymore!” went viral on the internet.
How did Sora achieve such a disruptive capability? This has to do with the two core technological breakthroughs behind it - Spacetime Patch technology and Diffusion Transformer (DiT) architecture.
NBD searched for the original papers of these two technologies and found that the Spacetime Patch paper was actually published by Google DeepMind scientists in July 2023. The first author of the DiT architecture paper was William Peebles, one of the leaders of the Sora team. But ironically, this paper was rejected by the Computer Vision Conference in 2023 for “lack of innovation”, and only a year later, it became one of the core theories of Sora.
Today, the Sora team has undoubtedly become one of the most watched technology teams in the world. The reporter checked the OpenAI official website and found that the Sora team was led by Peebles and two others, and the core members included 12 people, among whom there were several Chinese. It is worth noting that this team is very young, and has not been established for more than a year.
Core Breakthrough One: Spacetime Patch, Standing on Google’s Shoulders
Previously, OpenAI showed several cases of Sora transforming static images into dynamic videos on the X platform, which impressed people with their realism. How did Sora do this? This has to do with the two core technologies behind the AI video model - DiT architecture and Spacetime Patch.
According to foreign media reports, Spacetime Patch is one of the core innovations of Sora, which is based on Google DeepMind’s early research on NaViT (Native Resolution Vision Transformer) and ViT (Vision Transformer).
Patch can be understood as the basic unit of Sora, just like the basic unit of GPT-4 is Token. Token is a fragment of text, Patch is a fragment of video. GPT-4 is trained to process a string of Tokens and predict the next Token. Sora follows the same logic, it can process a series of Patches and predict the next Patch in the sequence.
Sora’s breakthrough lies in the fact that it treats video as a patch sequence through Spacetime Patch, and Sora maintains the original aspect ratio and resolution, similar to NaViT’s processing of images. This is crucial for capturing the true nature of visual data, allowing the model to learn from more accurate expressions, thus giving Sora near-perfect accuracy. As a result, Sora can effectively handle various visual data without any preprocessing steps such as resizing or padding.
NBD noticed that the Sora technical report released by OpenAI revealed the main theoretical basis of Sora, among which the Patch paper was titled Patch n’ Pack: NaViT, a Vision Transformer for any Aspect Ratio and Resolution. The reporter searched the preprint website arxiv and found that this research paper was published by Google DeepMind scientists in July 2023.
Photo/ arxiv.org
Photo/Google Scholar
Core Breakthrough Two: Diffusion Transformer Architecture, Related Paper Was Rejected
In addition, another major breakthrough of Sora is the architecture it uses. Traditional text-to-video models (such as Runway, Stable Diffusion) are usually diffusion models (Diffusion Model), text models such as GPT-4 are Transformer models, while Sora uses DiT architecture, which combines the characteristics of both.
According to reports, the training process of traditional diffusion models is to gradually add noise to the image through multiple steps, until the image becomes a completely unstructured noise image, and then in the image generation, gradually reduce the noise, until a clear image is restored. The architecture used by Sora is to process the input image containing noise through the Transformer’s encoder-decoder architecture, and predict a clearer image at each step. The DiT architecture combined with Spacetime Patch allows Sora to train on more data, and the output quality is also greatly improved.
The Sora technical report released by OpenAI revealed that the DiT architecture used by Sora was based on a paper titled Scalable diffusion models with transformers. The reporter searched the preprint website arxiv and found that the original paper was published by William (Bill) Peebles, a researcher at Berkeley University, and Saining Xie, a researcher at New York University, in December 2022. William (Bill) Peebles later joined OpenAI and led the Sora technology team.
Image source: arxiv.org
However, ironically, Meta’s AI scientist Yann LeCun revealed on the X platform that “this paper was rejected by the Computer Vision Conference (CVR2023) in 2023 for ‘lack of innovation’, but was accepted and published at the International Computer Vision Conference (ICCV2023) in 2023, and became the basis of Sora.”
Photo/X platform
As one of the most knowledgeable people about DiT architecture, after Sora’s release, Saining Xie posted some guesses and technical explanations about Sora on the X platform, and said, “Sora is indeed amazing, it will completely change the video generation field.”
“When Bill and I participated in the DiT project, we did not focus on innovation, but on two aspects: simplicity and scalability.” He wrote. “Simplicity represents flexibility. One of the often overlooked highlights of the standard ViT is that it makes the model more flexible in processing input data. For example, in the Masked Autoencoder (MAE), ViT helps us only process the visible blocks, ignoring the masked ones. Similarly, Sora can control the size of the generated video by arranging randomly initialized blocks in a suitable size grid.”
Photo/X platform
However, he believes that there are still two key points that have not been mentioned about Sora. One is about the source and construction of training data, which means that data is likely to be the key factor for Sora’s success; the other is about (autoregressive) long video generation, Sora’s one of the breakthroughs is being able to generate long videos, but OpenAI has not revealed the technical details.
Young Development Team: Fresh PhD Leads, and There Are Post-2000s With Sora’s popularity, the Sora team also came to the center of the world stage, attracting continuous attention. The reporter checked the OpenAI official website and found that the Sora team was led by William Peebles and two others, and the core members included 12 people. Judging from the graduation and entry time of the team leaders and members, this team was established for a short time, and has not been more than a year.
Photo/OpenAI official website
In terms of age, this team is also very young, and the two research leaders both graduated with PhDs in 2023. William (Bill) Peebles graduated in May last year, and his co-authored diffusion Transformer paper became the core theory of Sora. Tim Brooks graduated in January last year, and was one of the authors of DALL-E 3. He worked at Google and Nvidia.
Photo/William (Bill) Peebles personal homepage
There are even post-2000s among the team members. Will DePue, who was born in 2003, graduated from the University of Michigan Computer Science Department in 2022 and joined the Sora project team in January this year.
Photo/Will DePue personal homepage
In addition, there are several Chinese in the team. According to media reports, Li Jing is the co-first author of DALL-E 3. He graduated from Peking University’s Department of Physics in 2014, received a PhD in Physics from MIT in 2019, and joined OpenAI in 2022. Ricky Wang switched from Meta to OpenAI in January this year. The rest of the Chinese employees include Yufei Guo and others who have not yet been introduced in much public information.