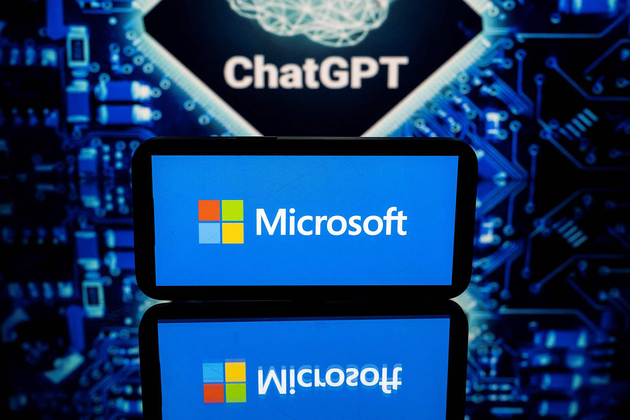
Photo/VCG
Artificial intelligence (AI) models rely on massive amounts of data to learn and improve their capabilities. However, as the demand for data increases, the supply of high-quality and accessible data becomes more limited and costly.
Some AI companies have started to use synthetic data, which is data generated by AI models that mimic real data, to train their systems. Synthetic data can offer advantages such as lower cost, higher diversity, and better privacy protection.
However, synthetic data also poses challenges and risks, such as the quality and reliability of the generated data, and the potential degradation of the content on the internet.
The New York Times bans AI development with its content
The issue of data scarcity was highlighted by a recent change in the terms of service of The New York Times, which stated that its news articles and images are prohibited from being used for AI development without prior written consent. This means that the high-quality data that tech companies can freely use to train their large language models (LLMs) is becoming more scarce.
LLMs are AI systems that can generate natural language texts for various applications, such as chatbots, voice assistants, and content creation. LLMs such as ChatGPT and Bard are trained by scraping data from the internet, including digitized books, news articles, blogs, search queries, X (formerly Twitter) and Reddit posts, YouTube videos, and Flickr images.
According to The Economist, tech giants Google and Meta (formerly Facebook) have trained their latest AI models on more than one trillion words each. By comparison, the online encyclopedia Wikipedia has about four billion English words.
Data is expensive
Data is expensive and competitive in the AI era. In 2018, Microsoft paid $7.5bn to acquire GitHub, a software code repository, as a dedicated data set for developing a code-writing AI tool.
As the demand for data grows, model builders are eager to find more new sources of data to maintain their frantic “feeding”. Companies that have large amounts of such data resources are weighing how to best profit from them, and they also have more bargaining power.
For example, Reddit and Stack Overflow have increased the cost of accessing their data. X has taken measures to limit the ability of bots to scrape its site, and now charges anyone who wants to access its data. According to Reddit’s official website, the free rate limit for using its data API is: only 100 queries per minute per client ID; if no client ID is used, only 10 queries per minute; if these limits are exceeded, a fee of $0.24 per 1,000 API requests is required.
Nevertheless, in order to obtain more data to train better LLMs, tech companies are willing to pay a high price.
In July, OpenAI signed a deal with The Associated Press. Recently, the company also expanded its deal with Shutterstock, a stock photography provider. Meta also reached a deal with Shutterstock. In August, Google was reported to be in talks with Universal Music Group to license artists’ voices to support AI song creation.
As the demand for data increases, start-ups also scrabbling to get a share of the market. In April, Weaviate, a database company focused on AI, raised $50m at a valuation of $200m; less than a week later, another data start-up PineCone raised $100m at a valuation of $750m; earlier this month, Neon raised another $46m.
Synthetic data offers new solution
Facing the dilemma of data shortage, Microsoft, OpenAI and Cohere have started to turn to synthetic data as a new solution. Synthetic data is data generated by AI models that mimics real data but is not exactly the same as real data. It is used to train other AI models.
For example, to train an advanced mathematics model, Cohere uses two AI models that talk to each other: one acts as a maths tutor and the other acts as a student. Humans act as supervisors and intervene and correct if the models say something wrong.
Cohere’s CEO Aidan Gomez said: “If you could get all the data you need from the internet, that would be great. But the reality is that the internet is so noisy and messy that it doesn’t really represent what you want. The internet can’t offer everything we need.”
Two studies from Microsoft Research show that using synthetic data to “feed” AI is feasible. For instance, using GPT-4-generated “children’s novel” dataset TinyStories which only contains words that four-year-olds can understand can still train a large model that can generate grammatically correct and fluent stories.
Another paper shows that AI can be trained by synthesising Python code and that these codes perform relatively well on coding tasks.
Image source: arXiv.org
Gomez pointed out that in order to improve LLMs’ performance and be able to cope with challenges in science, medicine or business, AI models will need unique and complex datasets. These data either have to be created by world experts such as scientists, doctors, writers, actors or engineers, or obtained from proprietary data from large companies such as pharmaceuticals, banks and retailers. “However, these human-created data are very expensive.” Synthetic data has a clear cost advantage because it does not require collecting and annotating real data.
New challenges emerge: synthetic data quality questioned
As the new trend of synthetic data emerges, start-ups such as Scale AI and Gretel.ai have sprung up, focusing on providing synthetic data services for tech companies. Among these companies, Gretel has received support from Google, HSBC, Riot Games and Illumina.
This means that more and more large companies are getting involved in the field of synthetic data.
However, although synthetic data seems promising, there are also criticisms that it cannot reflect or improve the real-world data. The quality and reliability of the synthetic data depends on the level and method of the AI model that generates it. If the generated data differs from or contains errors in the real data, then the trained model may also have problems.
As AI-generated texts and images begin to flood the internet, AI companies scraping training data on the internet may eventually inevitably use original data generated by their own models’ original versions - this phenomenon is called “dog-fooding”.
A recent study by universities such as Oxford University and Cambridge University called The Curse of Recursion: Training on Generated Data Makes Models Forget warns that training AI models based on their own original output (which may contain false or fabricated content) may over time damage and reduce technical performance, resulting in “irreversible defects”.
The paper’s author, Ross Anderson, professor of security engineering at Cambridge University and Edinburgh University, said: “Just as we have filled the oceans with plastic waste and the atmosphere with carbon dioxide, we are about to fill the internet with nonsense.”
Gretel’s CEO Ali Golshan also agreed: “As more and more content on the internet is generated by AI, I do think that over time this will lead to content degradation, because language models are producing repetitive knowledge without any new insights.”